Using Big Data Analytics for Transboundary Water Management
Southern Africa has experienced drought-flood cycles for the past decade that strain the ability of any country to properly manage water resources. This dynamic is exacerbated by human drivers such as the heavy reliance of sectors such as mining and agriculture on groundwater and surface water, as well as subsistence agriculture in rural areas along rivers. These factors have progressively depleted natural freshwater systems and contributed to an accumulation of sediment in river systems. In a region where two or more countries share many of the groundwater and surface resources, water security cuts across the socioeconomic divide and is both a rural and urban issue. For example, the City of Cape Town had to heavily ration all water uses in 2017 and 2018, as its dams were drying up.
New technology, however, brings new opportunities for improved water governance. In Southern Africa, university researchers and government agencies are joining with international development groups and the private sector to explore how big data analytics can improve the management of aquifers that are shared by two or more countries.
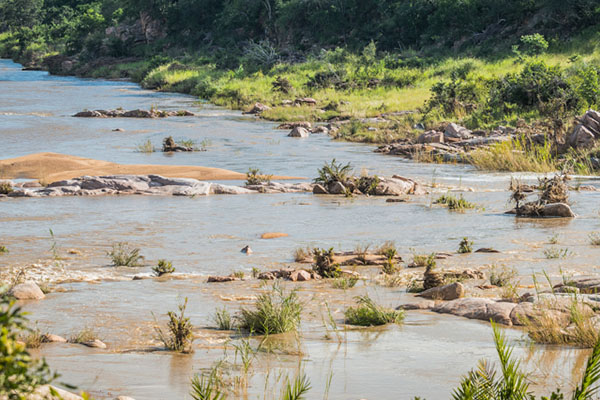
Initiated by the USAID Global Development Lab and IBM Research Africa, this effort, known as the Big Data Analytics and Transboundary Water Collaboration for Southern Africa, or the Collaboration, aims to improve water security by promoting big data approaches for regional collaboration. Partners in the Collaboration include the South African Department of Science and Innovation, the Water Research Commission of South Africa, the Southern Africa Development Community-Groundwater Management Institute, the USAID Southern African Regional Mission, the U.S. Geological Survey, and the USAID Center for Water Security, Sanitation, and Hygiene. USAID’s Sustainable Water Partnership (SWP) was tasked with providing technical leadership and coordinating four research teams focusing on the Ramotswa aquifer, which Botswana and South Africa share, and the Shire aquifer, which Malawi and Mozambique share.
Research underway addresses several topics, including data standardization, data availability, and data sharing between countries that share water resources, and the application of big data analytics to the resulting data sets. The research teams have the opportunity to leverage the expertise of IBM Research Africa to explore ways to use the latest technology to collect and analyze data to improve water resource management. Big data analytics will allow basin managers to collect and sift enormous amounts of data to analyze trends and patterns, and leverage artificial intelligence techniques such as machine learning to improve the management of the Ramotswa and Shire aquifers.
The lessons learned from this Collaboration will contribute to a digitization and data automation process initiated by the South African and Botswana Department of Water and Sanitation for water monitoring and smart decision-making. Ultimately it will help improve management of all shared aquifers in the 16 member states of the Southern African Development Community (SADC).
The Power of Machine Learning
Machine learning is a form of artificial intelligence. In the last decade, deep learning techniques, a subset of machine learning that mimics how the human brain sorts through data to make decisions, have begun to surpass human performance in recognizing images. A growth in computational power has facilitated the use of massive datasets generated by satellites, the increased use of social media and other web-based applications for daily life. Sophisticated algorithms have been created to process substantial amounts of data and return valuable information on consumer behavior, natural processes, trade and economics, and many other sectors.
“The general process for machine learning is that data gets fed into a model, and that model will make a prediction based on what it has seen,” said Naweed Khan, a research scientist with IBM Research Africa, in a webinar series coordinated by SWP on big data analytics and machine learning in the water sector. . “If that prediction is incorrect, then error signals will propagate from the prediction all the way back in order to identify what were the contributing factors to getting the prediction wrong.”
Transboundary Water Management
Big data techniques have been used in Southern Africa in recent years to identify methane contamination in groundwater, simulate the behavior of aquifers, and predict continuous groundwater level values. Still, local data on groundwater is often incomplete and comes in different formats depending on the country. The Collaboration is working to address the need to standardize data from disparate databases and structures, while researching new ways of analyzing and visualizing groundwater data through the four research projects.
Standardization means agreeing on how to collect and format water data. For instance, one country could measure water in liters while a neighboring country collects it in gallons. When dealing with large amounts of data, converting these metrics can result in huge mistakes. Another issue is how often data is collected. If one country collects data daily, while another collects only weekly, researchers can conduct only a weekly analysis. Finally, different countries often observe different methodologies when collecting water data, producing discrepancies in data quality.
While this research remains in progress, there are a couple early lessons. Stakeholders need a mutual shared interest, with each partner directly benefitting. For example, the IBM Africa Lab is getting the opportunity through the Collaboration to advance its technology by applying it to the water sector in Southern Africa. Local universities and their countries are benefitting, as students from partner universities are getting internships with IBM in Johannesburg.
Addressing transboundary issues requires leveraging of resources and cross-sectoral collaboration. The Collaboration’s partners agreed to co-fund the four research projects. This helped secure commitment from the partners and ensured that partners were working within common institutional agendas and toward shared research goals. Thus far the partners have worked together to find common ground between their institutional agendas and collaboratively find ways to finance their shared agenda.
To learn more about the potential of big data for transboundary water management, join the Collaboration’s LinkedIn group, or view previous webinars conducted by U.S. Geological Survey on “Tools and Methodologies for Data Analytics and Groundwater Management.”
This article was written by SWP’s Clara Bocchino and Knowles Adkisson, and originally published at newsecuritybeat.org.